Machine learning has become an essential tool for predictive analytics, allowing businesses to uncover valuable insights and make data-driven decisions. By utilizing algorithms and statistical models, machine learning can analyze vast amounts of data to predict outcomes and trends with a high degree of accuracy. In this article, we will explore the role of machine learning in predictive analytics and how organizations can leverage this technology to improve decision-making and drive business success.
1. Understanding the Basics: What is Machine Learning and Predictive Analytics?
Machine learning is a powerful tool that allows computers to learn from data and make predictions without being explicitly programmed. Predictive analytics, on the other hand, is the process of using data, statistical algorithms, and machine learning techniques to identify the likelihood of future outcomes based on historical data.
One key concept in machine learning is the ability to recognize patterns in data and make informed predictions based on those patterns. This is crucial for predictive analytics, as it enables businesses to anticipate trends and take proactive steps to capitalize on opportunities or mitigate risks.
By leveraging machine learning algorithms, businesses can analyze vast amounts of data to uncover insights and make accurate predictions about future events. This can help companies make more informed decisions, optimize processes, and ultimately drive success.
In the realm of predictive analytics, machine learning plays a vital role in identifying patterns, trends, and anomalies in data that human analysts may not be able to detect. This ability to extract valuable insights from data is essential for businesses looking to gain a competitive edge and stay ahead in today’s rapidly evolving market landscape.
2. Examining the Intersection of Machine Learning and Predictive Analytics
Machine learning has become a key tool in predictive analytics, transforming the way businesses make data-driven decisions. By analyzing large datasets and identifying patterns, machine learning algorithms can predict future outcomes with a high level of accuracy.
The intersection of machine learning and predictive analytics is where the magic happens. Machine learning algorithms, such as neural networks and decision trees, are applied to historical data to build predictive models that can forecast future trends and behaviors. These models continuously learn and improve over time, making them invaluable for businesses looking to stay ahead in today’s competitive landscape.
Incorporating machine learning into predictive analytics processes can uncover hidden insights and opportunities that traditional methods may overlook. Businesses can leverage these insights to make more informed decisions, reduce risks, and optimize operations for maximum efficiency.
By harnessing the power of machine learning, businesses can enhance their predictive analytics capabilities and drive success through data-driven strategies. Embracing this technology is essential for staying competitive in a rapidly evolving business environment.
3. The Role of Machine Learning in Enhancing Predictive Business Analytics
Machine learning plays a crucial role in enhancing predictive business analytics by utilizing algorithms and statistical models to analyze data patterns and make accurate predictions. One key advantage of machine learning is its ability to handle large volumes of data more efficiently than traditional analytical methods. By identifying complex patterns and trends within datasets, machine learning algorithms can provide valuable insights for businesses to make informed decisions.
Moreover, machine learning can adapt and learn from new data inputs, continuously improving its predictive capabilities over time. This adaptability allows businesses to stay ahead of market trends and make proactive decisions based on real-time data analysis. Additionally, machine learning can automate various processes, saving time and resources while improving the accuracy of predictions.
Incorporating machine learning into predictive analytics can lead to more accurate forecasting, better risk management, and enhanced decision-making for businesses across various industries. It is essential for companies to leverage the power of machine learning to unlock valuable insights and drive growth in today’s competitive business landscape.
4. Practical Applications of Machine Learning in Predictive Analytics
Machine Learning plays a pivotal role in the field of Predictive Analytics by leveraging algorithms and statistical models to analyze data and make informed predictions. In practical applications, Machine Learning algorithms can be used to identify patterns and trends within large datasets, enabling organizations to anticipate future outcomes accurately.
One of the key is in the healthcare sector. By utilizing predictive modeling, healthcare providers can anticipate patient outcomes, optimize treatment plans, and improve patient care. This not only enhances the quality of healthcare delivery but also reduces costs and improves patient satisfaction.
Machine Learning is also extensively used in financial services for fraud detection and risk assessment. By analyzing transaction data and customer behavior patterns, Machine Learning algorithms can identify fraudulent activities in real-time, helping financial institutions mitigate risks and protect their customers’ assets.
Furthermore, in marketing and sales, Machine Learning algorithms can analyze customer data to predict buying behavior, tailor personalized recommendations, and optimize marketing campaigns for higher conversion rates. This leads to increased customer engagement and loyalty, ultimately driving business growth.
Overall, Machine Learning is an indispensable tool in Predictive Analytics, revolutionizing decision-making processes and driving business success.
5. Embracing Machine Learning: Enhancing Predictive Analytics for Business Success
Machine learning plays a pivotal role in enhancing predictive analytics for businesses. By utilizing advanced algorithms and data analysis, machine learning models can analyze large datasets to uncover patterns and trends that humans may not easily identify. This leads to more accurate predictions and insights for informed decision-making.
One key advantage of machine learning in predictive analytics is its ability to adapt and learn from new data inputs. This dynamic learning process enables organizations to continuously refine their predictive models, staying ahead of changing market trends and customer preferences.
By harnessing machine learning for predictive analytics, businesses gain a competitive edge in forecasting customer behavior, optimizing marketing strategies, and enhancing operational efficiencies. This data-driven approach can boost revenue, improve customer satisfaction, and drive overall business success.
Incorporating machine learning into predictive analytics is not merely a trend but a necessary strategy for organizations looking to thrive in a data-driven world. The synergy between these two fields is invaluable for unlocking valuable insights and making informed decisions that drive business growth and innovation.
Conclusion
In conclusion, machine learning has proven to be a valuable tool for predictive analytics, allowing organizations to extract valuable insights from their data and make informed decisions. By leveraging advanced algorithms and powerful computing capabilities, businesses can gain a competitive edge in today’s data-driven world. As the technology continues to evolve, the potential applications of machine learning in predictive analytics are endless, promising even greater advancements in the field.
References:
1. ”Machine Learning and Predictive Analytics” by Eric Siegel (2016)
2. “Predictive Analytics: The Power to Predict Who Will Click, Buy, Lie, or Die” by Eric Siegel (2013)
3. “Machine Learning: The New AI” by Ethem Alpaydin (2019)
4. ”Predictive Analytics for Dummies” by Dr. Anasse Bari, Mohamed Chaouchi, and Tommy Jung (2016)
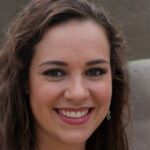
Karmen Grier is a versatile writer who effortlessly delves into diverse subjects, ranging from travel and lifestyle to health and wellness. With a warm and approachable tone, she invites readers to join her in exploring new experiences and finding balance in an ever-evolving world.